All Watched Over by Machines of Loving Grace
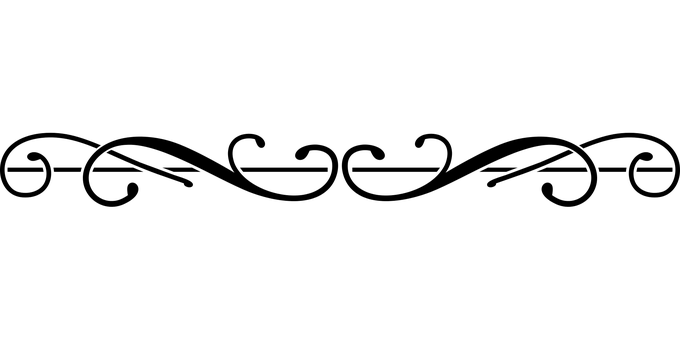
Introduction
All Watched Over by Machines of Loving Grace is a 1967 poem by Richard Brautigan that describes the peaceful and harmonious cohabitation of humans and computers.
You can read the poem or listen Richard Brautigan reading it:
At that time, this vision may have seemed very far away from a reality where there were only a few hundred computers around the world, each occupying an entire room but no more powerful than today's pocket calculator. Fifty years later, they are more than two billion computers, five billion smart phones and twenty billion IoT devices in the world. With the technological revolutions brought about by the internet, big data, the cloud and deep learning, Brautigan's vision resonates singularly and compel us to rethink our interactions with machines.
It is therefore not a coincidence that Adam Curtis used the title of Brautigan's poem for a three episodes documentary about how humans have been colonized by the machines they have built - "Although we don't realize it, the way we see everything in the world today is through the eyes of the computers." Curtis argues that computers have failed to liberate humanity, and instead have "distorted and simplified our view of the world around us".
You can see the first episode, the second and the last one:
The attack is severe but deserved. The potential wide-ranging impact urges to look carefully at the ways in which these technologies are being applied now, whom they’re benefiting, and how they’re structuring our social, economic, and interpersonal lives. The social implications of data systems, machine learning and artificial intelligence are now under scrutiny, with for instance the emergence of a dedicated research institute (AI Now). The massive amount of data needed to couple the human’s world and the machine’s world, and their automatic handling, poses an unprecedented threat to individual freedom, justice and democracy.
But, beyond the misuse of these technologies that could perhaps be regulated by law, our face-to-face encounters with machines bring about major anthropological changes when they address not only the physical world, but also our moods, emotions and feelings. The question of how our personalities and preferences are being shaped by our digital surroundings seems more pressing than ever. How are our tools shaping us at the heart of the most intimate of human beings: emotion, art and creation?
GAFA may turn to ethology to help advance artificial intelligence, self-driving cars, and more. We turn to artists and computer scientists who work with artists, to fuel our reflections and question them in two webinars on October 14 and 15, on how digital tools, especially artificial intelligence, are shifting aesthetic issues and transforming the artistic workflow, challenging the notion of authorship, disrupting education and opening up new creative dimensions.
Before giving a brief, subjective and partial history of the interactions between music and artificial intelligence we will present some work by our speakers. They will illustrate our discussion during the two webinars. The links between music and computer science have indeed existed since the birth of the latter. It is perhaps its abstract character —the nature of imitation that connects music to the world is still discussed by philosophers— that has attracted computer scientists. In any case, people tried to get a computer to write music long before they tried to make it paint.
The few examples given will show that we are far from being able to replace composers and musicians. But above all, why do it?
What musical needs are met by the use of AI tools? How does one make music progress by using these tools? Our hypothesis is that music is an extraordinary field of experience that allows us to imagine new uses and new interactions with machines, going well beyond a tool towards a creative companionship; and that these machines allow us to better understand and to elaborate or test new answers to artistic questions: how do we evaluate a work, what is its value, what is the difference between novelty and modernity, how do we teach an artistic practice, what do we transmit on this occasion, and, among the most enigmatic, what is an artistic choice.
Performances with Machines, October 15, 11:00 a.m. (Atlanta) / 5:00 p.m. (Paris)
Some work in the field by our speakers
Working Creatively with Machines
Camine Emanuele Cella (CNMAT - CU Berkeley)
Composer and computer scientist, Carmine develops (amongst other thing) Orchidea an automated system to help instrumentation and orchestration.Rémi Mignot (Ircam)
Rémi do researches about audio indexing and classification (MIR) at STMS lab. Since 2018, he has been responsible of researches on music information retrieval in the analysis-synthesis team.Nicolas Obin (Sorbonne Université - Ircam)
Nicolas is associate professor at the Faculty of Sciences of Sorbonne Université and researcher in the STMS lab where his work focuses on speech synthesis and transformations, conversational agents, deep fakes, and computational musicology. He is actively involved in artistic creation with many collaborations in films, music production, and sound designers.Alex Ruthman (NYU)
Alex is Associate Professor of Music Education & Music Technology, and the Director of the NYU Music Experience Design Lab (MusEDLab) at NYU Steinhardt where he creates new technologies and experiences for music making, learning, and engagement. Digital technologies have disrupted art education and in particular music education. They have the potential to make Creative Musical Expression more accessible to all.Jason Freeman (Georgia Tech)
Jason is a Professor of Music at Georgia Tech and Chair of the School of Music. His artistic practice and scholarly research focus on using technology to engage diverse audiences in collaborative, experimental, and accessible musical experiences.
Recently, Jason co-designed EarSketch, a free online learning platform that leverages the appeal of music to teach students how to code. Used by over 500,000 show how students combine music and coding to create expressive computational artifacts, and exemplify how machine learning will create even deeper connections between music and.
Performances with Machines
Jérôme Nika (Ircam)
Jérôme is researcher in human-machine musical interaction in the Music Representations Team / STMS lab at IRCAM. Through the development of generative software instruments, Jérôme Nika’s research focuses on the integration of scenarios in music generation processes, and on the dialectic between reactivity and planning in interactive human-computer/music improvisation. His work takes place in the broad family of Omax approaches to human-machine musical interactions.Benjamin Levy (Ircam)
Benjamin is a computer music designer at IRCAM. He collaborated on both scientific and musical project implying AI, in particular around the OMax improvisation software. The artistic project A.I. Swing marks several years of artistic and experimental experiences with musician and jazz improviser Raphaël Imbert.Grace Leslie (Georgia Tech)
Grace is a flutist, electronic musician, and scientist at Georgia Tech. She develops brain-music interfaces and other physiological sensor systems that reveal aspects of her internal cognitive and affective state to an audience.Daniele Ghisi (composer)
Daniele studied and composition. He is the creator, together with Andrea Agostini, of the project bach: automated composer’s helper, a real-time library of computer-aided composition. AI technics were instrumental in his work for La Fabrique des Monstres. His work explores many facets of the relationship between digital tools and music. In the installation An Experiment With Time, which can be viewed online from October 12 to October 18, is journey through three different time cycles, their dreams and the construction of a time-dilating machine.Elaine Chew (CNRS)
Elaine is a senior researcher and pianist at the STMS Lab and PI of the ERC projects COSMOS and HEART.FM. She designs mathematical representations and analytical computational processes to decode musicians' knowledge and explain artistic choices in expressive musical performance. She integrates her research into concert-conversations that showcase scientific visualisations and lab-grown compositions. She has collaborated with Dorien Herremans to create the MorpheuS system, and used algorithmic techniques to make pieces based on arrhythmia electrocardiograms.
Special online Event
From online from October 12 to October 18, the audience can access
An Experiment With Time, an audio and video installation by Daniele Ghisi
inspired by a book bearing the same name published by John W. Dunne, an aeronautical engineer and philosopher. John Dune believed that he experienced precognitive dreams and proposed that our experience of time as linear is an illusion brought about by human consciousness.
A central theme addressed in the installation of Daniele Ghisi is the construction and sharing of time and dreams between humans, and its transformation in the face of technology. If Richard Brautigan's vision comes true, how will we share our time with machines? How can we reconcile the elastic time of our human activities, from the dolce farniente to the ubris of our the Anthropocene era, and the regulated, chronometric, Procrustean time of the tireless machine?
A brief and subjective history of
AI technics in music composition
The Early Days
Electronic music i.e., music that employs electronic musical instruments, has been produced since the end of the 19th century. But producing a sound by a computer needed the existence of computers and the earliest known recording of computer music was recorded at Alan Turing's Computing Machine Laboratory in Manchester in 1951. Listen to the Copeland and Long's restauration of the recording and the account of the all-night programming session which led to its creation, and Turing's reaction on hearing it the following morning
In the late 1940s, Alan Turing noticed that he could produce notes of different pitches by modulating the control of the computer's loudspeaker used to signal the end of a calculation batch. Christopher Strachey used this trick to make the first pieces: the national anthem, a nursery rhyme and Gleen Miller's “In the Mood”.
By the summer of 1952, Christopher Strachey develop "a complete game of Draughts at a reasonable speed". He was also responsible of the strange love-letters that appear on the notice board of Manchester University’s Computer Department from August 1953.
Strachey's method of generating love letters by computer is to expand a template by substituting randomly chosen words at certain location. Locations belong to certain categories and each category corresponds to a pool of predefined words. The algorithm used by Strachey is as follows:
You are my" Adjective Noun2. "My" Adjective(optional) Noun Adverb(optional) Verb, Your Adjective(optional) Noun
Generate "Your" Adverb, "MUC"
Algorithmic control is in italic, locations (placeholders) are underlined and fixed sequence in the output are in bold.
It is the same process that was used in the 18th century by the Musikalisches Würfelspiel to randomly generate music from precomposed options. One of the earliest known examples are the Der allezeit fertige Menuetten– und Polonaisencomponist proposed in 1757 by Johann Philipp Kirnberger. Here is an example by the Kaiser string quartet:
Carl Philipp Emanuel Bach used the same approach in 1758 to propose Einfall, einen doppelten Contrapunct in der Octave von sechs Tacten zu machen, ohne die Regeln davon zu wissen (German for "A method for making six bars of double counterpoint at the octave without knowing the rules"). A perhaps better-known example is that of W. A. Mozart's Musikalisches Würfelspiel K.516f Trio 2 proposed here by Derek Houl:
At the time, people chose at random using a dice. In 1957, a computer was used: Lejaren Hiller, in collaboration with Leonard Issacson, programmed one of the first computers, the ILLIAC at the University of Illinois at Urbana-Champaign, to produce what is considered the first score entirely generated by a computer. Named Illiac suite, it later became the String Quartet number 4.
The piece is a pioneering work for string quartet, corresponding to four experiments. The two composers, professor at the University, explicitly underline the research character of this suite, which they regard as a laboratory guide. The rules of composition and order that define the music of different epochs are transformed in automated algorithmic processes: the first is about the generation of cantus firmi, the second generates four-voice segments with various rules, the third deals with rhythm, dynamics and playing instructions, the fourth explores various stochastic processes:
Whether in musical dice games or in the Illiac suite, a dialectic emerges between a set of rules driving the structure and form of a piece, and the randomness used to ensure a certain diversity and the exploration of an immense combinatorial game. This dialectic is at work in almost every automated composition system.
At the same time, in France, Iannis Xenakis was also exploring several stochastic processes to generate musical material. He will also mobilize other mathematical notions to design new generative musical processes. In his first book, Musiques formelles (1963; translated in English with three added chapters as Formalized Music – Thought and mathematics in composition, 1972), he previews for instance the application to his work of probability theory (in the pieces Pithoprakta and Achorripsis, 1956-1957), ensemble theory (Herma, 1960-1961) and game theory (Duel, 1959; Stratégie, 1962).
Expert systems and symbolic knowledge representation
We jump in time to the eighties. Expert systems are flourishing. This set of technics takes a logical approach to knowledge representation and inference. The idea is to apply a set of predefined rules to facts to produce a reasoning or answer a question. These systems have been used to generate scores by explaining rules that describe a musical form or the style of a composer. The rules of fugue, or Schenkerian analysis, for example, are used to harmonize in the style of Bach.
A notable example of the rule approach is given by the work of Kemal Ebcioğlu at the end of the eighties. In his PhD thesis work (An Expert System for Harmonization of Chorales in the Style of J.S. Bach) he develops the CHORAL system based on 3 principles:
![]() |
|
---|
Backtracking is a technique used in particular for constraint satisfaction problems, which allows a series of choices to be questioned when these choices lead to an impasse. For example, f we build a musical sequence incrementally, it may happen at some point that we can no longer increment this sequence without violating the constraints we have set ourselves. The idea is then to go back to a previous point of choice and make another choice to develop an alternative. If there are no further possible choices, one has to go back to the previous choice point, and so on until one can develop a complete solution.
Heuristics are practical methods, often relying on incomplete or approximate knowledge, which do not guarantee correct reasoning, but which often produce satisfactory results (and quickly). When the search for an optimal solution is not feasible nor very practical, heuristic methods can be used to speed up the process of finding a suitable solution.
Here is an example of chorale harmonization (first the orignal Bach’s harmonization then teh result produced by CHORAl at 4’42). The concert note skeches the expert system:
Another outstanding example from the same decade is the EMI system “Experiment in Music Intelligence” developed by David Cope at the University of Santa Cruz. David Cope began to develop this system while he was stuck on writing an opera:
“I decided I would just go ahead and work with some of the AI I knew and program something that would produce music in my style. I would say ‘ah, I wouldn’t do that!’ and then go off and do what I would do. So it was kind of a provocateur, something to provoke me into composing.”
https://computerhistory.org/blog/algorithmic-music-david-cope-and-emi/
The system analyzes the pieces submitted to it as input characterizing a “style”. This analysis is then used to generate new pieces in the same style. The analysis of EMI applied to his own pieces, makes the composer aware of his own idiosyncrasies, of his borrowings and finally leads him to make his writing evolve:
“I looked for signatures of Cope style. I was hearing suddenly Ligeti and not David Cope.” the composer noted, “As Stravinski said, ‘good composers borrow, great composers steal’. This was borrowing, this was not stealing and I wanted to be a real, professional thief. So I had to hide some of that stuff, so I changed my style based on what I was observing through the output [of] Emmy, and that was just great.”
https://computerhistory.org/blog/algorithmic-music-david-cope-and-emi/
You can hear many pieces produced by this system. Listen at a A Mazurka in the style of Chopin produced by EMI, and an intermezzo in the manner of Mahler.
Right from the start, David Cope wanted to distribute this music in the classic commercial circuit. They are often co-signed with Emmy, the little name that designates his system. Over the years, the system has evolved with sequels called Alena and Emily Howell who is also a recorded artists.
When David Cope is asked if the computer is creative, he answers :
“Oh, there's no doubt about it. Yes, yes, a million times yes. Creativity is easy; awareness, intelligence, that's hard.”
GOFAI versus Numerical Approaches
Subsequent versions of EMI also use learning techniques that blossomed again in the early 2000s. As a mater of fact, throughout the history of computer science, two approaches have confronted each other.
Symbolic reasoning denotes the AI methods based on understandable, explicit and explainable high-level "symbolic" (human-readable) representations of problems. Knowledge and information is often represented by logical predicates. The preceding examples fall more into this category. The term GOFAI ("Good Old-Fashioned Artificial Intelligence") has been given to symbolic AI in the middle of the eigthies.
During this decade, there was a return to the forefront of a range of digital techniques, often inspired by biology but including also advances in statistical sciences and in numerical machine learning. Machine learning relies on numerical representations of the information to be processed. An example of a technique that falls within this domain are artificial neural networks. This technique was already used in the 1960s with the perceptron invented in 1957 by Frank Rosenblatt which allows supervised learning of classifiers. For Instance, a perceptron can be trained to recognize the letters of the alphabet in handwriting. The input of the system is a pixel array containing the letter to be recognized, and the output is the recognized letter. During the learning phase many examples of each letter are presented and the system is adjusted to produce the correct output categorization. Once the training has been completed, a pixel array can be presented containing a letter that is not part of the examples used for training and the system correctly recognizes the letter.
Depending on the time, the dominant paradigm in AI has fluctuated. In the sixties, machine learning was fancy. But at the end of the decade, a famous article put the brakes on this field, showing that perceptrons could not classify anything. This was because its architecture was reduced to a single layer of neurons. It is shown in the following that more complex classes of examples can be recognized by increasing the number of neuron layers. Unfortunately, there was no learning algorithm available at that time to train multi-layered networks.
Such an algorithm appeared in the 1980s but it is still very heavy to implement and it is also realized that to train a multi-layer network, you need a lot, a lot of data.
Machine Learning
At the beginning of the 2000s, the algorithms are still making progress, the machines are much faster and we can access numerous databases of examples as a result of the development of all digital techniques. This favorable conjunction relaunched numerical machine learning techniques and we now encounter the term deep learning at every turn (here “deep” refers to the many layers of the network to be trained).
The contribution of these digital learning techniques is considerable. It allows for example to generate sound directly and not a score (the sound signal being much richer in information, it takes many layers to do this and hours of recorded music to train the network). We have examples of instrument sounds reconstructed by these techniques.
Bass | Glockenspiel | Flugelhorn | |
---|---|---|---|
original | |||
Wavenet |
Of course, one can also compose, and there are many examples of Bach's choir. Here is an example of an organ piece produced by a neural network (folk-rnn) and then harmonized by another (DeepBach). And another example of what can be achieved (with folk-rnn) by training a network on 23,962 Scottish folk songs (from midi type transcriptions).
One challenge faced by machine learning is that of the learning data. For reasons that are rarely discussed, and despite all academic and non-academic researches, the project of interpreting music is a profoundly complex and relational endeavor. Music is a remarkably slippery things, laden with multiple potential meanings, irresolvable questions, and contradictions. Entire subfields of philosophy, art history, and media theory are dedicated to teasing out all the nuances of the unstable relationship between music, emotion and meanings. The same question haunts the domain of images.
The economic stakes are not far away. A company like AIVA thus organized a concert (at the Louvre Abu Dhabi) featuring five short pieces composed by their system and played by a symphony orchestra. Other examples include a piece composed especially for the Luxembourg national holiday in 2017. and an excerpt from an album of Chinese music:
But beware, in fact only the melody is computer generated. The orchestration work, arrangements, etc., are then done by humans: https://www.aiva.ai/engine. This is also true for a lot of systems that are claiming automatic machine composition, including Schubert's Unfinished Symphony No. 8, finished by a Huawei smartphone.
From automatic composition to musical companionship
Making music automatically with a computer is probably of little interest to a composer (and to the listener). But the techniques mentioned can be used to solve compositional problems or to develop new kind of performances. An example in composition is to produce an interpolation between two rhythms A and B (given at the beginning of the recording)
Another compositional example is to help orchestration problems. The Orchid* software family, initiated in Gérard Assayag's RepMus team at IRCAM, proposes an orchestral score that comes as close as possible to a given target sound as input. The latest iteration of the system, Orchidea, developed by Carmine Cella, composer and researcher at the Univ. of Berkeley, gives not only interesting but also useful results. Some (short) examples are available on the page:
An original archeos bell and its orchestral imitation
A girl’s and an orchestra’s screaming
Falling drops and the orchestral results
A roaster and it musical counterpart
Far from a replacement approach, where AI substitutes for human, these new techniques suggest the possibility of a musical companionship.
This is the objective of the OMax family of systems, developed at IRCAM, still in Gérard Assayag's team. OMax and its siblings have been performed all around the world with great performers and numerous videos of public performances and concerts demonstrates the system's abilities. These systems implements agents producing music by creatively navigating a musical memory learned before or during the performance. They offer a wide range of ways to be "composed" or "played".
An example conceived and developed by Georges Bloch with Hervé Sellin at the piano, to which Piaf and Schwartzkopf respond on the theme of The Man I Love. The second part of the video presents a reactive agent that listen to saxophonis Rémi Fox and play back previous musical phrases recorded live during the same performance:
In this other example, a virtual saxophone dialogues with the human saxophonist in real time following the structure of a funk piece. The latest version of OMAX developed by Jérôme Nika, researcher at Ircam, combines a notion of composed "scenario" with reactive listening to drive the navigation through the musical memory. In the three short excerpts, the system responds by reacting to Rémi Fox's saxophone by focusing
- first on timbre (beginning – 28s),
- then on energy (29s – 57s),
- and finally on melody and harmony (58s – end)
The type of music generation strategy used to co-improvise in the last example, was also used for Lullaby Experience, a project developed by Pascal Dusapin using llulabies collected from the public via the Internet. There is no improvisation here. The system is used to produce material which is then taken up with the composer and integrated with the orchestra.
A last example where AI assists the composer rather than substituting for her or him, is given by La Fabrique des Monstres by Daniel Ghisi. The musical material of the piece is the output of a network of neurons at various stages of its learning on various corpuses. At the beginning of the learning process, the music generated is rudimentary, but as the training progresses, one recognizes more and more typical musical structures. In a poetic mise en abîme, the humanization of Frankenstein's creature is reflected in the learning of the machine:
A remarkable passage is StairwayToOpera which gives a "summary" of great moments typical of operatic arias.
As a temporary conclusion
These examples show that while these techniques can make music that is (often) not very interesting, they can also offer new forms of interaction, open new creative dimensions and renew intriguing and still unresolved questions:
How could emotional music be coming out of a program that had never heard a note, never lived a moment of life, never had any emotions whatsoever? (Douglas Hoffstader)
Jean-Louis Giavitto
CNRS – STMS lab, IRCAM, Sorbonne Université, Ministère de la Culture
giavitto@ircam.fr
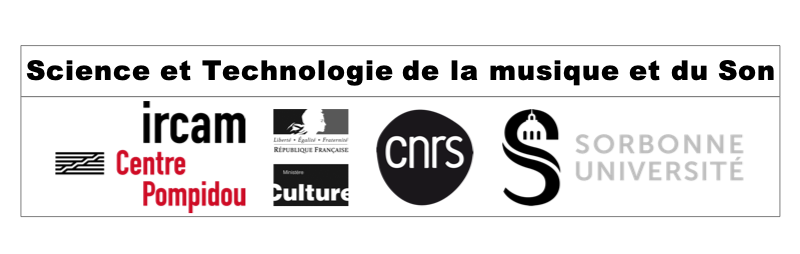